TABLE OF CONTENTS
Experience the Future of Speech Recognition Today
Try Vatis now, no credit card required.
Speech-to-text technology is an AI-powered solution that converts spoken words into written text. This technology is increasingly used in various domains, including healthcare, media, and finance, to transcribe audio and video content into text for analysis and storage purposes.
In this report, we compare Vatis Tech's accuracy to two of the biggest players in the market - Google and Speechmatics - and the results are impressive. In addition, the report showcases Vatis Tech's accuracy and highlights how it outperforms its competitors in various domains. Keep reading to dive into the details of this benchmark report and discover why Vatis Tech is the top choice for accurate and reliable speech recognition.
Dataset
In our benchmark report, we compared the latest version 4 of Vatis Tech, Speechmatics, and Google using a dataset of 45 minutes of audio from 6 domains, including:
- news
- financial calls
- podcasts
- medical
- legal
- meetings
The dataset was created to reflect the diversity of speech-to-text use cases and the challenges faced in each domain, and it ensures that the performance of the speech recognition solutions is tested in a range of real-world scenarios taking into account factors such as the speaker's accent, background noise, and word ambiguity.
Each domain has unique characteristics, such as accents, vocabulary, audio quality, and speech patterns, that can affect the accuracy of a voice-to-text converter. By including various audio content from these domains in the dataset, our benchmark accurately assessed the solution's ability to transcribe audio to text in different environments and with varying complexities.
Methodology
To compare the performance of three voice-to-text solutions: Vatis Tech, Speechmatics, and Google, we used 10 audio samples in the Romanian language from six domains: news, financial call, podcast, medical, legal, and meeting. This benchmark aimed to evaluate each speech-to-text converter's transcription accuracy and identify any strengths and weaknesses in their performance.
- Data Collection
The audio content used in this benchmark was collected from publicly available sources and included recordings of 45:33:00 minutes. More details about each piece of content from each domain are available in the dataset section of this report.
2. Evaluation Metrics
To evaluate the performance of the solutions, we used the following:
- a human transcription with 100% accuracy
- the word error rate (WER) metric
The WER is defined as the percentage of words in a transcription that are incorrectly transcribed by the solution technology. It represents the ratio of errors in a transcript to the total words spoken.
3. Performance Evaluation
To evaluate the performance of our speech-to-text solution, we utilized the standard metric Word Error Rate (WER). WER measures the accuracy of an automatic voice recognition system by comparing automated transcription with human transcription.
The WER score is derived from the Levenshtein distance, working at the word level, and is calculated based on the number of insertions, deletions, and substitutions made by the technology and is expressed as
(S + D + I) / N
where:
- S is the number of substitutions;
- D is the number of deletions;
- I is the number of insertions;
Before calculating the WER, we've applied a few normalization steps for each file to have both the reference (human transcriptions) and the automated transcriptions in the same format.
The normalization steps were:
- remove punctuation;
- lowercase all text;
- keep the formation of the numbers because we would like to evaluate the post-processing layer at the same time.
As an example, let's consider the WER score calculation for the following text:
- Reference: Hi, my name is Adrian, and I am 27 years old.
- Normalized reference: hi my name is adrian and i am 27 years old
Also, let's have a look at an example of a WER score calculation:
- Reference: Hi, my name is Adrian, and I am 27 years old.
- Normalized reference: hi my name is adrian and i am 27 years old
- Prediction: Hi, my name is Adrien, and I am 27 years old.
- Normalized prediction: hi my name is adrien and i am 27 years old
Thus the WER (normalized_reference, normalized_prediction) = 0.0909, which means the speech-to-text system's accuracy is 90.91%.
If you're interested in playing more with this metric, you can use the Hugging Face calculator from here.
Results
Let's take a closer look at a concrete example using the medical_sample_2 dataset. The transcript was initially transcribed with 100% accuracy by a human:
Tegumente cu aspect normal ecografic regiune mamelonară și retroaerolară cu aspect normal țesut mamar cu aspect fibrogramdular heterogen în cadranul inferior deschide paranteza ora 6 închide paranteza o formațiune nevascularizată, de 6,8 mm transversal slash 3,1 mm anteroposterior, cu aspect de fibro adenom paraleolar deschide paranteza ora 5 închide paranteza două formațiuni cu aspect de fibro adenom find delimitate între ele de 5 mm slash 3,7 mm și de 5,4 mm slash 3,6 mm la periferia glandei deschide paranteza ora 11:30 închide paranteza o formațiune de 5,9 mm slash 3,5 mm nevascularizată, cu aspect de fibro adenom fără dilatații ale canalelor galactofore țesut adipost spre și retromamar normal reprezentat lobulație păstrată cu aspect normal.
Here is the automated transcript generated by Vatis Tech:
Tegumente cu aspect normal ecografic regiune mame lonară și retroaerolară cu aspect normal țesut mamar cu aspect fibrogramdular heterogen încadra anul inferior deschide paranteza ora 6 închide paranteza o formațiune nevascularizată, de 6,8 mm transversal slash 3,1 mm anteroposterior, cu aspect de fibro-adenom paraleolar deschide paranteza ora 5 închide paranteza două formațiuni cu aspect de fibră adenom find delimitate între ele de 5 mm slash 3,7 mm și de 5,4 mm slash 3,6 mm la periferia glandei deschide paranteza ora 11 30 închide paranteza o formațiune de 5,9 mm slash 3,5 mm nevascularizată, cu aspect de fibră. Adenom fără dilatații ale canalelor galactofore țesutadipost spre și retromamar normal reprezentat lobulație păstrată cu aspect normal.
Speechmatics:
Tegument cu aspect normal ecografic, regiune mamă lunară și retro, aerul cu aspect normal țesut mamar cu aspect fibro glandular heterogen. În cadranul inferior. Deschide paranteza. Ora 6 Închide paranteza. O formațiune N vascularizată de 6,8 milimetri, transversal. Slash 3,1 mm. Antero posterior, cu aspect de fibro adenom. Paralele. Olar. Deschide paranteza. Ora 5 Închide paranteza. Două formațiuni cu aspect de fibro adenom fiind delimitate între ele de 5 mm slash 3,7 mm și de 5,4 mm. Flesh 3,6 mm la periferia glandei. Deschide paranteza. Ora 11:30 Închide paranteza. O formațiune de 5,9 mm slash 3,5 mm n. Vascularizată, cu aspect de fibro adenom. Fără dilatații ale canalelor galactofore. Țesut adipos pre și retro mamar normal, reprezentat globular e păstrat cu aspect normal.
and Google:
tegumente cu aspect normal ecografic regiune mamelonar și retro aerul ară cu aspect normal țesut mamar cu aspect fibro glandular heterogen în cadranul inferior deschide paranteza ora 6 închide paranteza o formațiune ne va scular is atât de 6,8 mm Trans lăsam Clash 3,1 mm anteroposterior cu aspect de fibroadenom paralel ar deschide paranteză ora 5:00 închide paranteza două formațiuni cu aspect de fibroadenom fiind delimitate între ele de 5 mm Slash 3,7 mm și de 5,4 m și 3,6 mm la periferia glandei deschide paranteza ora 11:30 închide paranteza o formațiune de 5,9 m m și 3,5 m m m vascularizată cu aspect de fibroadenom fără dilatații ale canalelor galactofore pe suta de post spre și retro mamar normal reprezentat lui Bula ție păstrată cu aspect normal
In this instance, we achieved an impressive 96.20% accuracy, while Speechmatics 80.80% and Google only 66.20%.
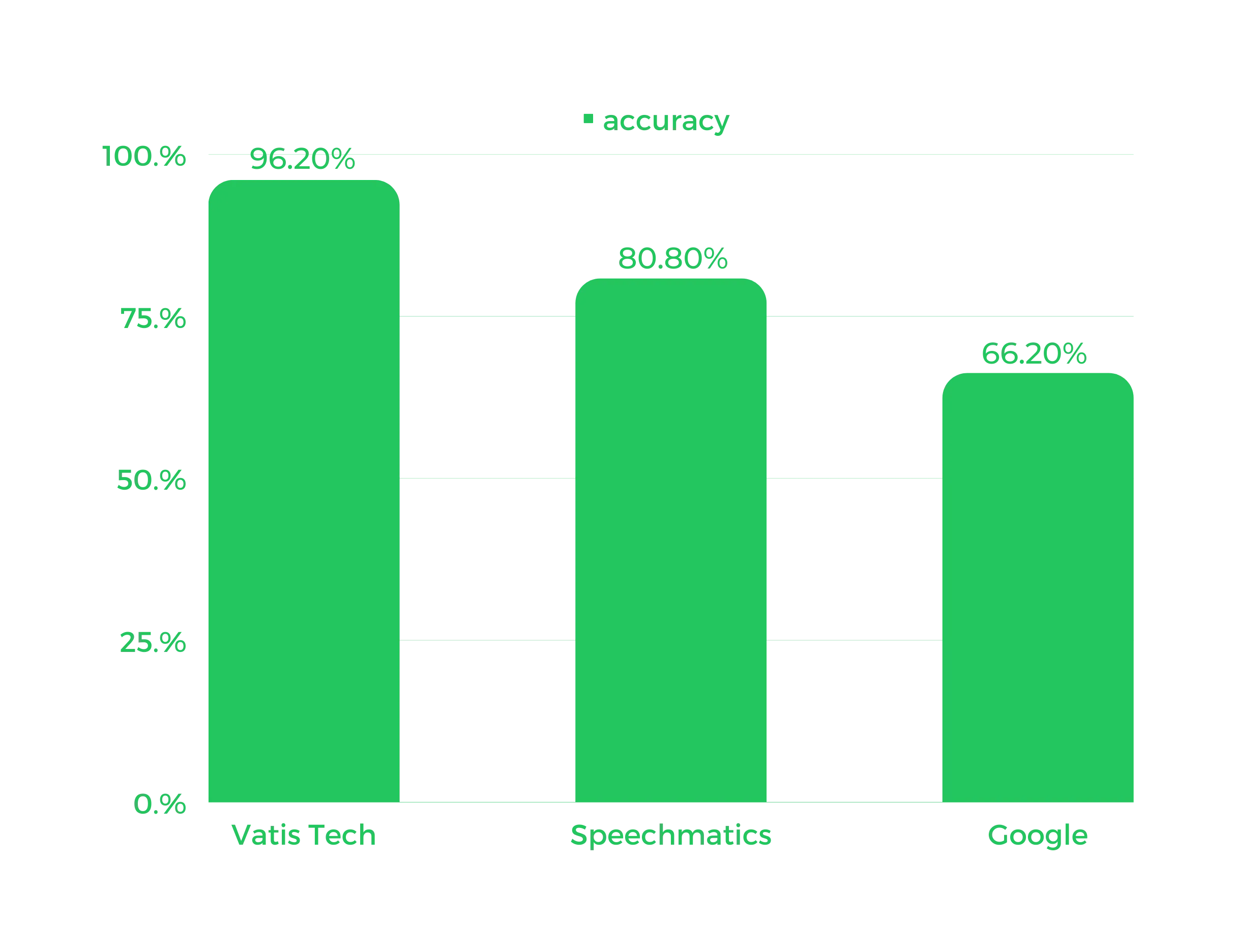
The results showed that Vatis Tech achieved an average accuracy of 95.30% for all datasets, while Speechmatics had an average accuracy of 89.39% and Google 68.80%. These results demonstrate the superior performance of Vatis Tech in converting speech-to-text, particularly in challenging domains such as medical, with an accuracy of 96.85%, and legal, with 98.10%.

Conclusion:
Our benchmark report comparing Vatis Tech, Speechmatics, and Google's latest audio to text converters has demonstrated the effectiveness of Vatis Tech's v4 model architecture. With an average accuracy of 95.30% across various audio domains, Vatis Tech has shown to be a leader in the field of voice recognition and transcription technology.
This report highlights the importance of using a diverse dataset to evaluate the performance of speech recognition solutions. The results provide valuable insights for both industry professionals and consumers seeking a high-quality voice to text converter.
Overall, the benchmark report has confirmed that Vatis Tech's v4 model architecture offers a cutting-edge solution for transcribing speech into text, making it a valuable asset for various industries and applications.